Distributed Reinforcement Learning for Autonomous Cooperation
Overview
Reinforcement learning provides a promising way to learn cooperation from interaction each robot. We study the problem of how robots can learn to cooperate with each other without a central controller and instigate the challenges of learning in this setting such as partner modeling, partial observations, large-scale cooperation and reward assignments. To address these challenges, we have proposed hierarchical reinforcement learning approach with partner modeling strategies. Our method learns a policy network that predicts the action-value and partner modeling networks that inferred the partners' strategy. The policy network is trained with distributed reinforcement learning, while the partner modeling networks are trained with supervised learning. To evaluate our approach, we have built a fundamental research testbed with high-realistic simulator and RL-friendly API.
Project Framework
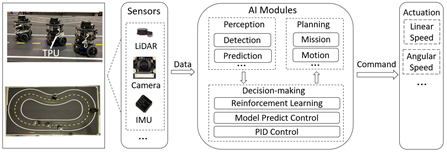
Demo
Achievements
- A silver medal at 2021 China (Shanghai) International Innovation and Invention Exhibition
- Zhixuan Liang, Jiannong Cao, Jinlin Chen et.al, SMART: Scalable Multi-robot Reinforcement Learning Platform, submitted to IEEE Internet of Things Journal
- Wang, Jia, et al. "Pattern-RL: Multi-robot Cooperative Pattern Formation via Deep Reinforcement Learning." 2019 18th IEEE International Conference On Machine Learning And Applications (ICMLA). IEEE, 2019
Members
Zhixuan Liang, Jinlin Chen, Huafeng Xu.
Previous Member includes Dr. Jia Wang. Thanks for the contributions.