Multi-stage Big Data Analysis on Complex Systems
Overview
Recently, big data has expanded rapidly and its applications in areas such as logistics, transportation and finance continue to grow, thereby taking a central position in our economy and society. Existing big data analytic methods are generally single-stage methods, meaning that they treat the system under study as an atomic entity with all its data directly available for analysis. However, most systems under study are complex, consisting of separable but interdependent subsystems, which are difficult to handle by existing single-stage methods. This brings new challenges and opportunities in all phases of data analytics including data collection, feature learning, model learning and application. In this project, we focus on developing new methodologies for multi-stage big data analytics on complex systems that address the challenges brought up by the diverse requirements, high dependency and correlated objectives of the subsystems. Specifically, we target at investigating four major tasks: 1) joint data collection; 2) joint feature learning; 3) joint model learning; and 4) the application of the proposal in the food supply chain system. This collaborative research is expected to generate new methodologies for multi-stage big data analysis on complex systems. An application demo will also be developed in the food supply chain system for performance evaluation through analyzing various food safety issues.
Project Framework
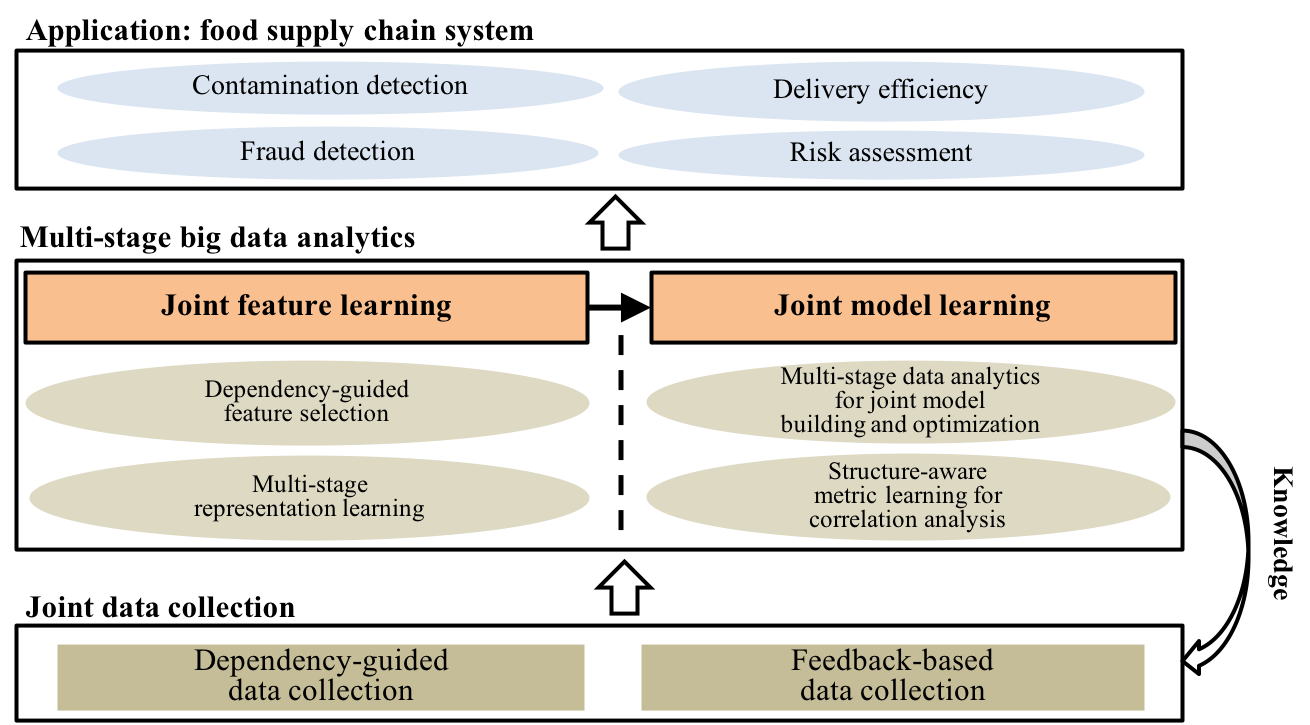
Members
Dr. Jiaxing Shen, Dr. Linchuan Xu, Dr. Wanyu Lin, Yu Yang, and Yuqing Zhao.
Previous members include Dr. Jia Wang and Dr. Senzhang Wang. Thanks for their contributions.