Learning Analytics based on Multilayer Behavior Fusion
Overview
Learning analytics is the measurement, collection, and analysis of data about learners and their contexts for the purposes of understanding and optimizing the process of learning and the underlying environment. Due to the complex nature of the learning process, existing works mostly focus on the modeling and analysis of single learning behavior and thus bears limited capacity in achieving good performance and interpretability of predictive tasks. We propose a research framework for learning analytics based on multilayer behavior fusion which achieves significantly better performance in various tasks including at-risk student early prediction and learning performance prediction. Results of extensive evaluation on thousands of students demonstrate the effectiveness of multilayer behavior fusion.
Early Prediction of At-risk Students with Online and Offline Learning Behaviors
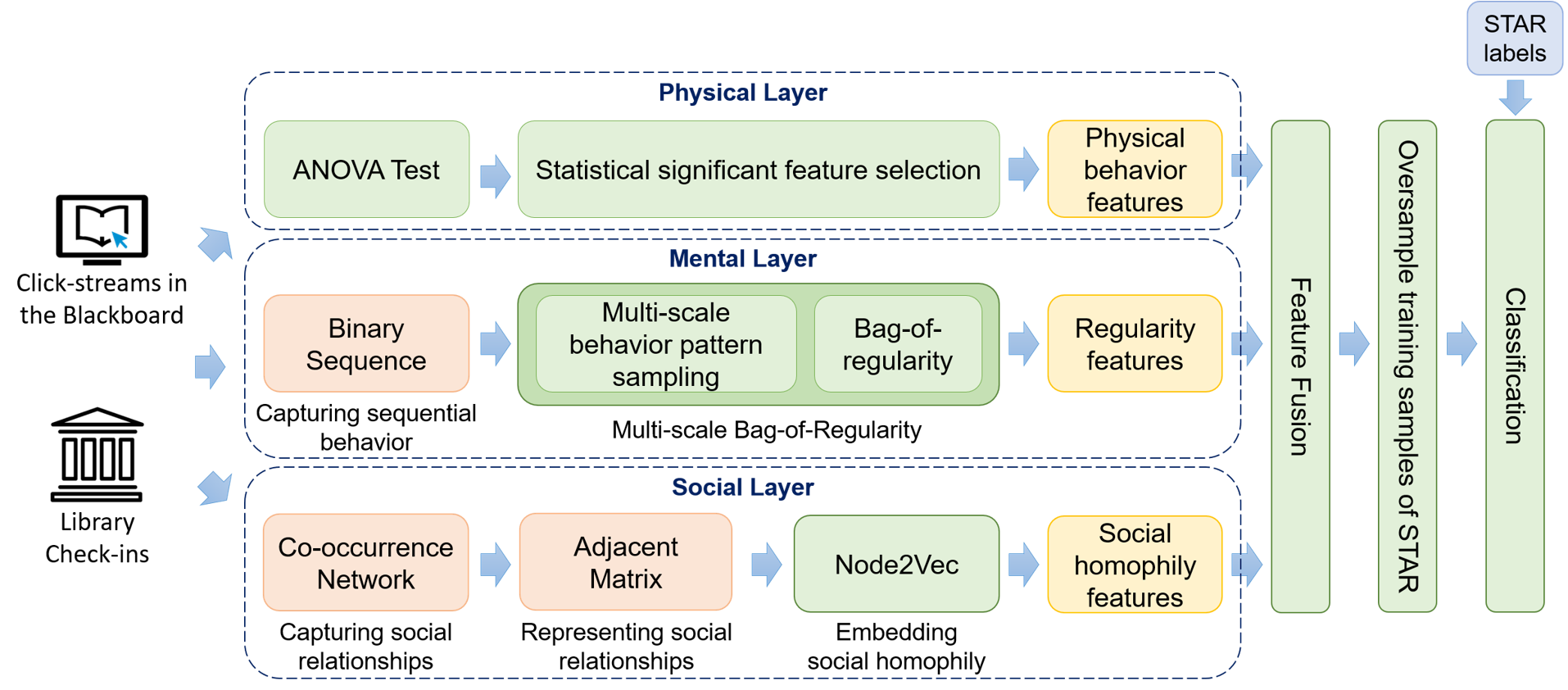
Early prediction of students at risk (STAR) is an effective and significant means to provide timely intervention for dropout and suicide. Existing works mostly rely on either online or offline learning behaviors which are not comprehensive enough to capture the whole learning process and lead to unsatisfying prediction performance. We propose a novel algorithm that could early predict STAR in a semester by modeling online and offline learning behaviors. The online behaviors come from the log of activities when students use the online learning management system. The offline behaviors derive from the check-in records of the library. Our main observations are two folds. significantly different from good students, STAR barely have regular and clear study routines. We devised a multi-scale bag-of-regularity method to extract the regularity of learning behaviors that is robust to sparse data. Second, friends of STAR are more likely to be at risk. We constructed a co-occurrence network to approximate the underlying social network and encode the social homophily as features through network embedding. The results indicate our approach outperforms baselines by 14.62% ~ 38.22% in predicting STAR.
Learning Performance Prediction from Online Forum Discussion
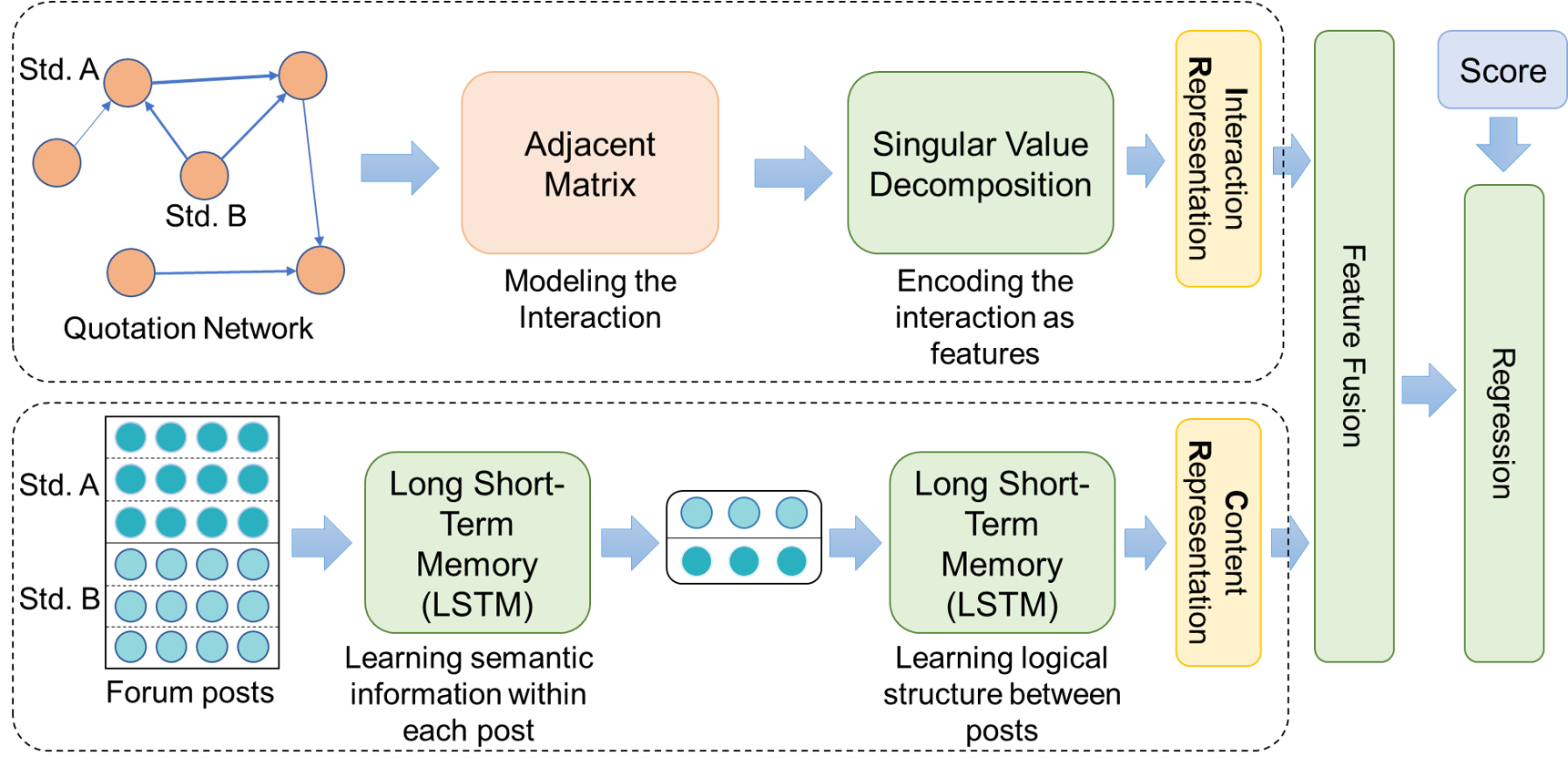
Online forum discussion is an effective and widely adopted means for instructors to facilitate online teaching. However, reading all the posts in the online forum and assess students' knowledge understanding and writing skills is extremely time-consuming and high labor cost. In this work, we aim to predict students' learning performance from their online discussion posts on given topics. We model the semantic consistency between posts and topics as well as utilize supporting arguments extracted from quoted posts as auxiliary posts representations. Specifically, we propose a mixture model including a hierarchical text model to measure the writing quality, a semantic matching model to model topic relevance, and a semantic representation model to integrate quoted posts. Compared to measuring writing quality alone, experimental results demonstrate that incorporating relevance could improve the performance of APS by a large margin.
Achievements
- Yu Yang, Zhiyuan Wen, Jiannong Cao, Jiaxing Shen, Hongzhi Yin, and Xiaofang Zhou EPARS: Early Prediction of At-risk Students with Online and Offline Learning Behaviors. DASFAA 2020: 3-19
- Yu Yang, Jiannong Cao, Jiaxing Shen, Ruosong Yang, and Zhiyuan Wen: Learning Analytics based on Multilayer Behavior Fusion. ICBL 2020: 15-24
Members
Dr. Jiaxing Shen, Yu Yang, Ruosong Yang, and Zhiyuan Wen.
Previous members include Dr. Shailey Chawla, Yiran Cheng, Yun Teng, Zhengyun Liao, Victor Cheng Wai-Tat. Thanks for their contributions.